Glaura da Conceicao Franco
Universidade de Minas Gerais, Brasil
Local: ZOOM – Link – password: 805991
03 Novembro 2021 (4.ª feira) – 14h:30m
Abstract:
Environmental epidemiological studies of the health effects of air pollution frequently utilize the generalized additive model (GAM) as the standard statistical methodology, considering the ambient air pollutants as explanatory covariates. Although exposure to air pollutants is multi-dimensional, the majority of these studies considers only a single pollutant as a covariate. This model restriction may be because the pollutant variables do not only have serial dependence but also interdependence between themselves.
In an attempt to convey a more realistic model, we propose here the hybrid generalized additive model – principal component analysis (PCA) – vector autoregressive model (VAR). The PCA is used to eliminate the multicollinearity between the pollutants whereas the VAR model is used to handle the serial correlation of the data to produce white noise processes as covariates in the GAM. Some theoretical and simulation results of the methodology proposed are discussed, with special attention to the effect of time correlation of the covariates on the PCA and, consequently, on the estimates of the parameters in the GAM and on the relative risk. As a main motivation to the methodology, a real data set is analysed with the aim of quantifying the association between respiratory disease and air pollution concentrations, especially particulate matter PM10, sulphur dioxide, nitrogen dioxide, carbon monoxide and ozone. The empirical results show that our model can remove the auto-correlations from the principal components. In addition, this method produces estimates of the relative risk, for each pollutant, which are not affected by the serial correlation in the data.
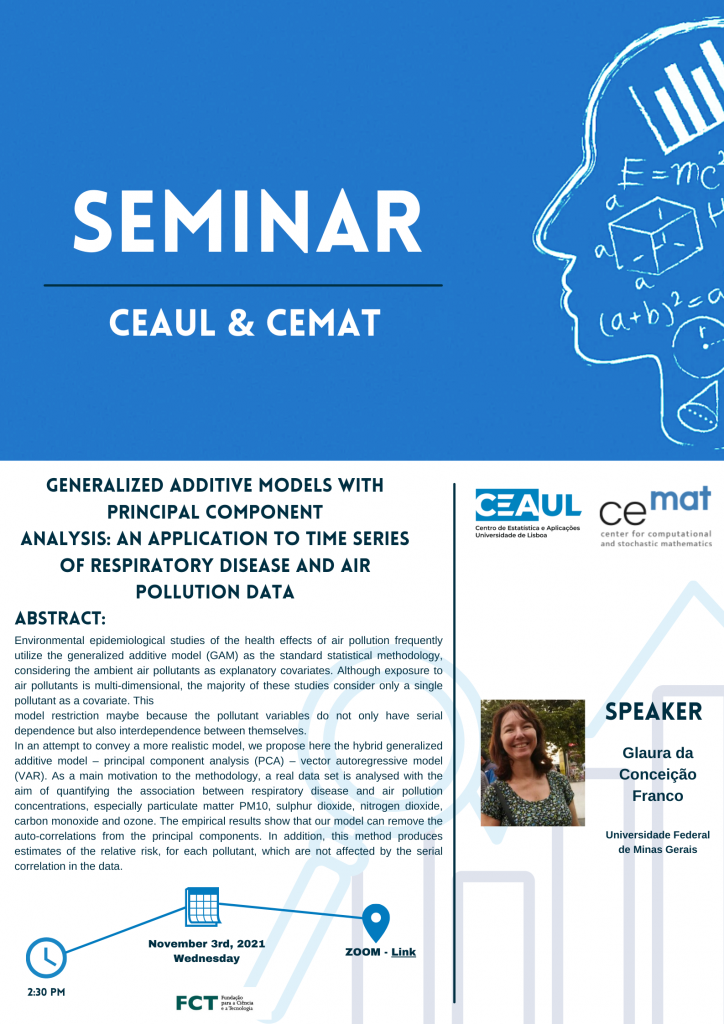